Innovation roulette tables from LP to OJSC
Even the ancient Greeks placed great emphasis on two things: philosophy and the enjoyment of life. Of course, they had time to think, compute, celebrate, and did not have to compete globally. What do we need today from their knowledge? What is certain is that there is no progression without innovation. As there is no innovation without open, accepting and inclusive thinking along with productive debate. Ergo, innovation could not exist without debate culture. In addition, it is very difficult to lure innovation without motivation. However, innovation is needed to stay in international competition, from shoemaker to irrigation equipment manufacturer, from teacher through lawyer to design engineer.
It is already an innovation if we copy others cleverly within frameworks: actually, it is not a basic research, but it can be a fruitful solution. This copy is well-known for Best Practices or industry-specific applications such as Good Manufacturing Practices (GMP). If we go on this path further, we have the option of reverse engineering, that is, decrypting the given device or software in order to ensure compatibility with our own device or software. The question of "ownership" of data is increasingly an issue - it is quite clear in the case of unique databases that it is covered by intellectual property protection. However, in cases where my machine provides data (e.g. temperature value) on industry standard protocol so that it is not possible to read it secondly (for example, because the bus system only supports one master), then the interfacing could be allowed for the reader processing machine installed by another authorized by the original manufacturer. The question is where and how the data is generated. There are a lot of manufacturers (we call them industry) who specialize in deconstructing tools and simply copying them cost-effectively - so we have to deal with them because they have to devote a fraction of the research costs to producing the products.
What is essential for innovation? We need brainstorming and basket of ideas from where we can draw from, and after 9 bad ideas we are expecting the 10th good idea to redeem, which we then try to translate into a business case or business plan. For good ideas and business case making you need to seeing the problem and have to have creativity, critical or improving thinking, time, experience (or on the contrary, virgin hands), viticulturalist, emotional intelligence should be needed the most. In terms of a business case, when we do not have a green light yet, it is particularly important that management understands and supports our business case, whether it is a project outside its field of expertise or not. It is vitally important for innovation, for example, that for an IT project, all the non-IT managers involved know the project, understand the project and see how it can improve its own field. Emotional intelligence needs to be addressed in order to unspoken fears, misunderstandings and feelings are treated not only at the moment of the decision, but throughout the entire duration of the innovation project. It is also necessary to enjoy the work. Those who do not enjoy their work or any aspect of it (e.g. to work in a good team), we cannot expect innovation from them. Do we need operators to innovate and bring new ideas? Obviously, if we don't want to stand at least 4 hours a day next to the machines as a leader. Is it possible to innovate in 8 hours or 3 shifts a day? How should we divide work and family life? There is a widespread belief that a "work-life balance" should be created, i.e. the balance between work and private life. According to recent schools and generations, this is almost impossible and worth thinking about in "work-life coexistence", i.e. in working / life co-operation / co-existence where it is not possible to separate sharply when work is done and when there is privacy. For example, before dinner, I write to someone quickly because of a tomorrow meeting, but after half a minute I have dinner (in fact, I can put the cutlery on the table with my left hand and send the message with my right hand). Unfortunately, or not, innovation requires that the workplace should go beyond, and work on the company's problems after work. If the employees have to worrying about a daily living, family, etc., there will be no free thoughts for the company, and they will even think about their privacy issues during their working hours and create their own private innovations at their workplace. It is therefore important to enable our employees to integrate innovation time into everyday thinking with a complex motivational package (relieving the burden). It is necessary to enjoy life for innovation - it is not possible to innovate by force.
Which innovation is good, which one is worthwhile, which one will make the most, or which will ensure the survival of the company? Some say it is unpredictable, roulette tables, others believe the business case is decisive, yet others believe in management buy-in with the support of the business case (called commitment only). Sure, if we act quickly, we'll quickly find out if the project was successful. If you try and prove the feasibility of an innovation idea with a small investment and a quick pilot project (PoC, the Proof of Concept), you can quickly move on to that innovation with a roll-out phase or switch to the next innovation idea. That's why we recommend focusing on a fast return (up to a few months) PoC within a year. What goes beyond this is more basic research or strategic development. In the case of innovations, if we want to rely on best practices, we can expect the following projects - based on our research - in the next 1-3 years:
- cyber security
- IoT
- multi-cloud environments
- Artificial Intelligence (AI)
- data processing analytics
- storage solutions
- Augmented Reality / Virtual Reality / Mixed Reality (AR / VR / MR)
- blockchain
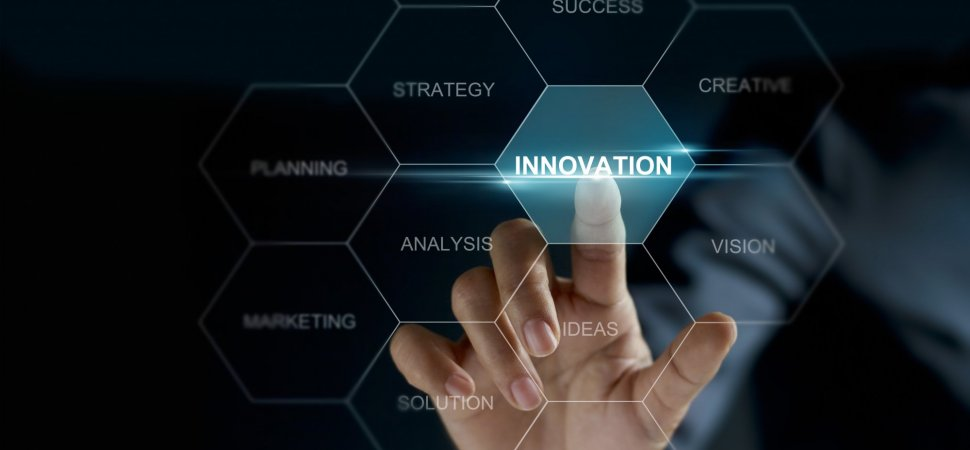
Of these, we would highlight the artificial intelligence (AI) that has been used in machine learning since the 1980s. Now, with the great AI flare up, “only” a multilevel neural network has been opened up to teach and quickly apply (deep learning) with the latest GPUs, that is, we have reached a level of speed and opportunity. We would like to draw attention to an important thing, which is very important for the protection of innovation: we can modify (or discard, if it goes in the wrong direction) the results (experiences) of machine learning and deep learning by further teaching, but it is difficult or impossible to copy or remove parts. In order for us to have a good solution for AI, we need "data philosophers" ("data artists" in other words) who, depending on the industry, understand processes, industrial goals, data, and are willing to think about what the difference between a pen and a brick if we can draw with both on the wall? When the customer's qualitative and quantitative expectations are so high that the state space and the variables cannot be tracked and managed either in the head, in the paper or in the spreadsheet, then the AI, for who this part is a child's play, is to be taught "only" to play correctly.
There is space and opportunity to innovate for every company, be it the basic infrastructure to provide the right work-life coexistence, relieve the employee of the unnecessary administrative burden by online methods or even by monitoring the production and environmental parameters based on more than 100 parameters to decide on the processes in real time. Small companies can innovate just like the biggest companies, in the least cases the best answer is to buy a new production machine. The world seems to drift in the direction that every company is basically an IT company (shoemakers and grocery stores, too), and such a class or a professionally oriented background class gives the company a special taste.
Tags :
IOT
CDR
Data
Process
Company
Projects
Innovation
Motivation
The essential role of the Local Hero in IoT projects
Where are we heading?
Thanks to the digitization of production, we’re in the midst of a significant transformation regarding the way we manufacture products. This trend points to a rise in automation, as well as the need for product teams to leverage the latest technology more than ever. There’s no doubt about it: We’re entering a new world of industry.
Many organizations might still be in denial about how Industry 4.0 could impact their businesses. Some are struggling to find the talent or knowledge to know how to best adopt it to their unique use cases. However, several others are implementing changes today and they are preparing for a future where smart machines improve their businesses.
What are Industry 4.0 and IoT about?
In spite of this, the greatest challenges companies face when building out their IoT capabilities do not lie in technology. Industry 4.0 and Internet of Things (IoT) are not really only about Machines. In fact, these are about people and transformation. If synergy is achieved with people, we will be just a few feet away from success.
Change management focuses on preparing and supporting the transformation of organisations at the level of individuals, teams and organisations as a whole. Nowadays, many of the change management processes are grafted into models, each with an own strategic approach and inspired by the experience of their developers.
How to get through the transformation smoothly?
In spite of the multiplicity of models, the underlying goal always remains the same: to guide the company and its employees smoothly through the transformation. In order to boost any change management process, there is a secret sauce: the key to success is the appointment of local heroes. These are individuals within a company who respond to changes more quickly than others.
Who will be the local hero?
During an Industry 4.0 change process, we always work in tandem: an external consultant, teamed up with an internal colleague. It is not just a few 'strangers' talking about how employees should do their work, but it is always the impact and influence of a familiar face.
This internal colleague is the so called 'local hero'. This person knows everything about the company, its processes and operations very well. The local hero has influence on events, has his/her own decision-making power and even knows the operational details. In terms of factories, it is typically a middle manager of production, maintenance, engineering or quality assurance who is aware of the goals to be achieved or the problems to be solved. As the result of the collaboration, the local hero has the chance to leave a huge impact on the IoT process.
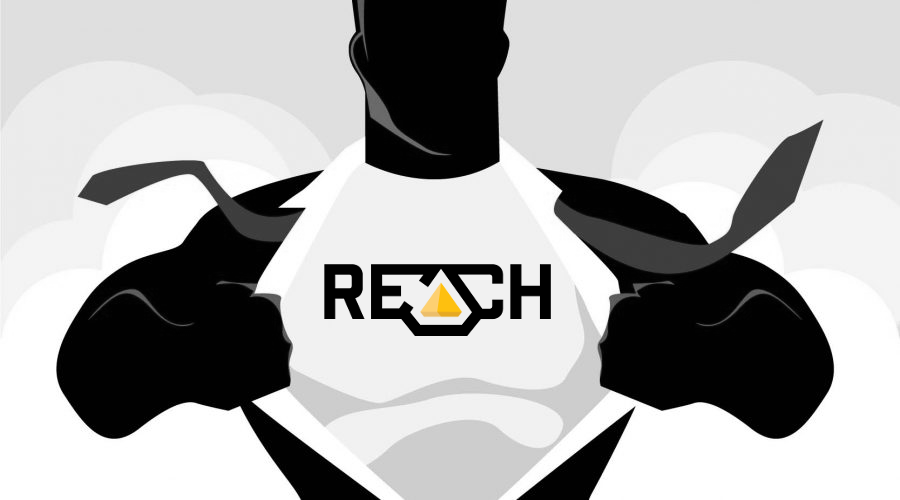
„You don’t create the Internet of Things as a stand-alone. Its value will come when it connects to all parts of the organization. That will lead to the transformation of the entire organization.” /Umeshwar Daya/
What is in the focus of innovation?
At the centre of the innovation that is unfolding across all geographic, industrial and technological borders are not so much those devices that are being linked together, but the 'connected person.' At the centre, there is a human being who is making use of the applications and services that are enabled by such devices and their unprecedented integration provided in the IoT.
Currently, machines are operated by humans and these machines only passively follow the operators' commands. The main trend of Industry 4.0 will therefore replace this condition by the Prognostics-monitoring system. Production processes will have to allow effective production.
At the same time, they should be flexible due to changing customer demand for particular products. The role of the human factor will be necessary for future manufacturing. The skills and qualifications of the workforce will become the key to the success of a highly innovative factory.
A local hero can make a huge difference here with a future-oriented, human-based attitude where everybody is involved and has its own inner motivation for a bright and common goal. Last but not least, this contribution also has a positive effect on the local hero's career path.
What does CDR stand for?
In this context, we can transfer CDR (Cloudera-DELL-REACH I4 reference architecture) abbreviation as a Career Development Revitalisation. A dedicated colleague will have the chance for a life-changing career development.
CDR will most likely offer a sky rocketing career path in the near future for the local hero. Nonetheless, CDR provides unique experience for the local hero, which will make this colleague precious in the eye of the company. Most importantly, however, the local hero can use the acquired knowledge and vision at a higher managerial level.
Hidden value of diagnostic data
PLC - an alternative solution instead of traditional assembly lines
Among the numerous challenges in Industry 4.0/ Industrial Internet of Things (IIoT) solutions, the most intensely discussed and developed area is probably the pursuit for a continuous, precise and efficient production process. In order to increase Key Performance Indicators (KPI) to the highest possible levels, manufacturers must pay attention to obtaining machine operational data analyzed in a transparent, yet interrelated method.
The abilities of cutting-edge diagnostic tools are increasingly linked to AI/ ML methods. According to Gartner, by 2020, over 40% of tasks related to data analysis will be totally automated. It is no surprise as human data analysis skills are not very agile .
However, some programmable logic control (PLC) vendors already offer machine-fault detecting algorithms through built-in libraries. These solutions help improve production line uptime by providing engineers with machine health assessment. This is a useful tool to prepare preventive maintenance (PVM) before an accidental component failure causes an unexpected system downtime incident.
On the other hand, due to changing customer habits, factories need to be able to respond to personalized product needs. Thus, they should make their production more flexible towards smaller batches than before. Along with this approach, factories will set up innovative and flexible manufacturing areas with interconnected automation islands instead of the traditional fixed assembly lines.
Obtaining elementary machine data
Without any doubt, the greatest bottleneck of IIoT and I4.0 is data transfer between layers that have never communicated with each other before. The number of solutions here corresponds to the number of vendors. Here, a common goal is to substitute classical 'package-sending' and find real-time data extraction methods from the memory area of controllers without any radical intervention.
Industrial robots mostly use Real-Time Data Exchange (RTDE), RESTful API's that leverage the HTTP protocol; and the messages are composed of XHTML and JSON, etc. The situation is not any better with PLC's, where you can find MC protocol, S7, Modbus, Ethernet/IP FINS/TCP, OPC UA, MQTT and so on.
To handle communication challenges between different kinds of systems, machines and devices, we need to create standardized protocols, interfaces and data-interchange formats. Moreover, different devices can serve different sample rates (0.2…500 Hz). This results in different data volumes and densities with different timestamps. REACH's event-based smart data lake is specialized for the fast interpretation of such disorderly data streams.
Motion diagnostics
Since the third industrial revolution, the market of industrial robots has been growing in an unstoppable way. Today, as a result of this enormous growth, industrial robots including their diagnostics are in the absolute focus of attention. Robotic arms are mostly preferred because of their manipulative abilities. They are able to move their tools quickly and accurately. This works even with high payload and in a variety of applications.
Special AC servo motors are responsible for moving the individual joints to the correct position. Monitoring their status and diagnosing motion patterns can provide elementary information on their proper functioning.
Under normal operating conditions, motors in a robotic arm work within a current value (disturbance) interval pre-defined by the manufacturer (depending on current inertia). They return to their positions within a given pulse coder error. The motion torque applied to an arm must be cyclical.
With the help of modern IIoT connectors, we can observe cycle patterns by collecting the mechanical parameters of robotic arms. Inappropriate or missed maintenance, collision and mechanical abnormalities can be detected through discrepancies in these patterns. Restrictions to factory limit values or a machine learning algorithm may be appropriate.
For example, with a properly configured notification management system, an overcurrent (OVC) alarm and downtime can be prevented. An escalation process is triggered by the first signs of an anomaly. At the same time, the maintenance staff is immediately informed of the exact technical details and the list of components required for intervention, as well as of the estimated remaining availability.
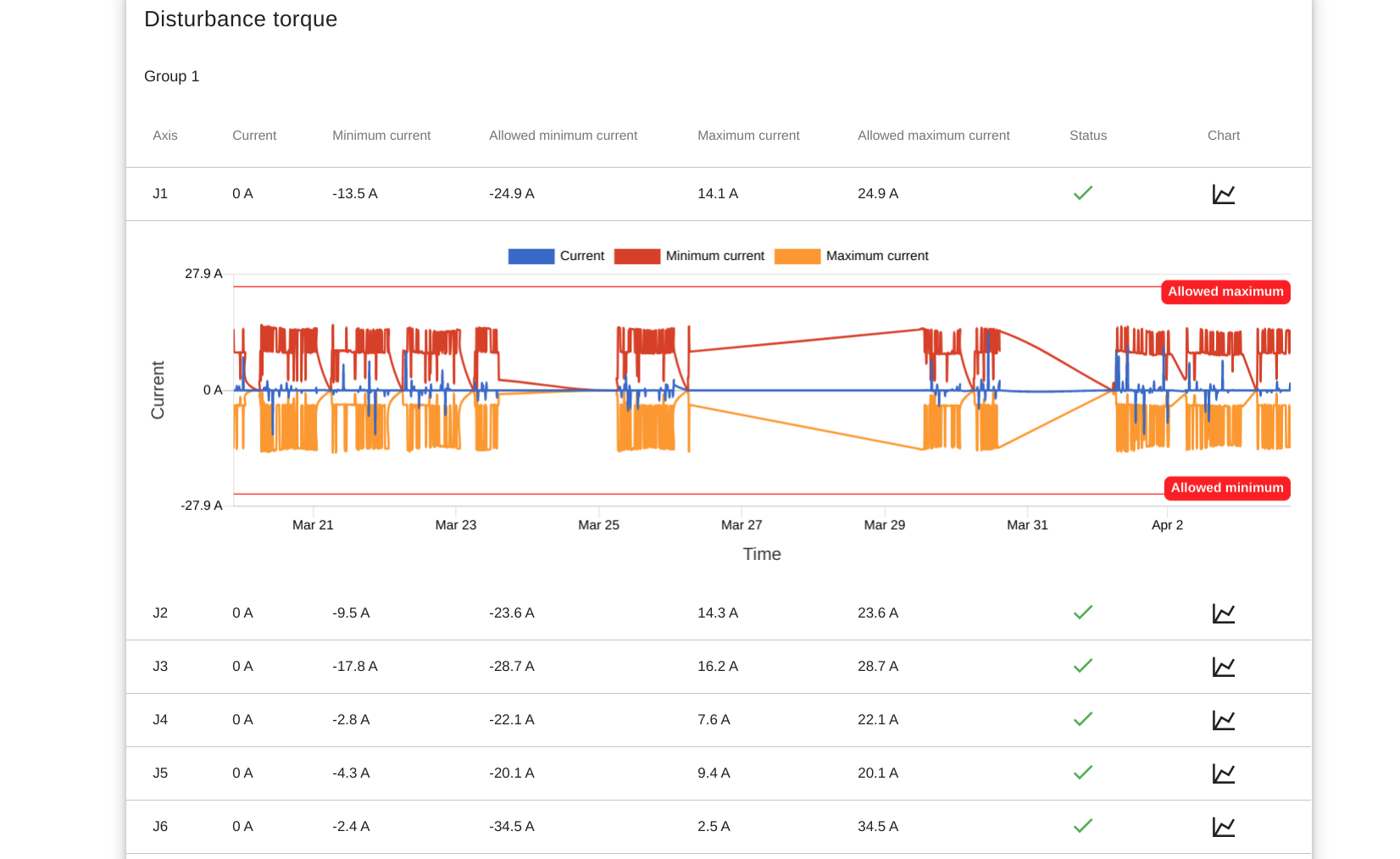
Error rates and heatmap
Statistical and predictive tools are based on historical smart data lakes and they cover a wide range of mechanical diagnostics. Time and quantity diagnostics of each error event bring the sequential phenomena into focus. When investigating the advantages and disadvantages of sequential phenomena analysis, we can conclude that it can be served with a relatively small hardware resource requirement for a properly designed database of its computing layers. However, it does not handle or highlight parallel events. This is helped by a so-called correlation heat map, which is a tool for investigating correlations between data series.
The outcome of the analysis is greatly influenced by the quantity and quality of the data. If all data sources from the manufacturing process are integrated into the architecture, the complex phenomena can be examined and solved on a unique analytical interface. This learns the relationship between the tool status and the number of scraps or stoppage causes of the machine, as well as material or operator failure, or even tool failure with the available algorithms.
Key to the correlation heat map below: values close to 1 (red) have a strong positive correlation (time-time movement), while values close to -1 (blue) show a strong negative correlation (time-opposing change).
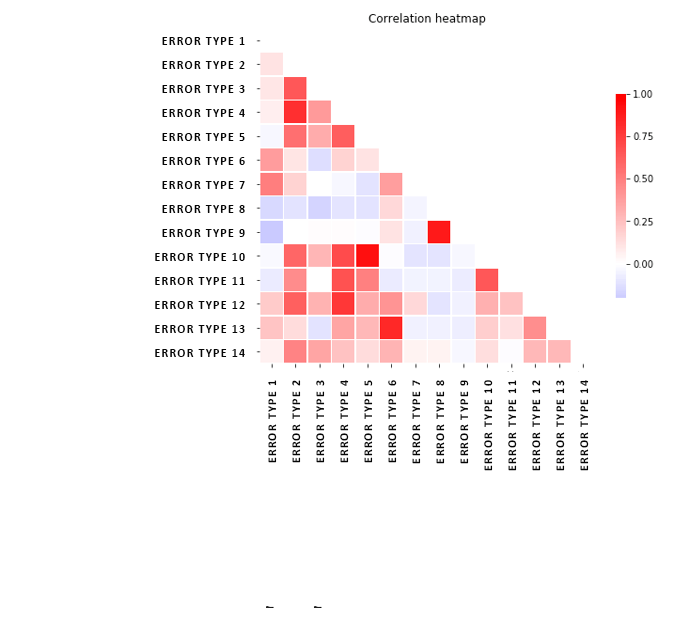
Conclusion
The above approaches have both direct and indirect feedbacks to the production process. In both cases, a high resolution interface with the existing MES is essential. To ensure continuous production, the proper scheduling of necessary maintenance is a simple, yet important formula to recycle machine diagnostic data. In case of small series production, we can override any previous production schedule based on the diagnostic data obtained. In case of a predicted failure, we can save precious downtime by optimizing the sequence of series by reorganizing the production between the islands.
All these can be created in an autonomous environment, with machine-to-machine (M2M) communication via standard channels (e.g. OPC UA), without human interaction.
Application layer protocols
Key aspects of Internet of Things (IoT) and Industry 4.0 are sending and receiving data. To handle communication between different kinds of systems, sensors and devices, we need to have standardized protocols. These protocols should be a little bit different from the currently used protocols over the internet.
Another great example is one from the application side. Consider the classic HTTP/HTTPs based data access and try to cover a modern real-time application with simple HTTP requests, this is not an option. That is where we need to use new type of protocols.
In our current blog posts we will introduce the new protocol definitions for the TCP/IP definition application layer.
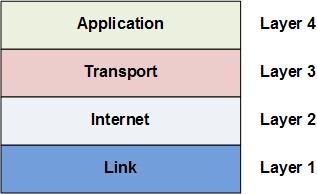
REST - Representational State Transfer
Representational State Transfer is an architectural style that build on certain principles using the current web fundamentals. It generally runs on HTTP. It makes a stateless transfer. REST ignores the details of component implementation and protocol syntax in order to focus on the roles of components, the constraints upon their interaction with other components, and their interpretation of significant data elements. REST has been applied to describe desired web architecture, to identify existing problems, to compare alternative solutions, and to ensure that protocol extensions would not violate the core constraints that make the Web successful. Fielding used REST to design HTTP 1.1 and Uniform Resource Identifiers (URI).
The REST architectural style is also applied to the development of web services as an alternative to other distributed communication types such like SOAP. REST is often used in mobile applications, social networking Web sites, mashup tools and automated business processes.
There are 5 basic fundamentals of REST services which are created for the Web.
- Everything is a Resource.
- Every Resource is Identified by a Unique Identifier.
- Use Simple and Uniform Interfaces
- Communication is Done by Representation.
- Every Request is Stateless.
WS - Websocket
The HTML5 WebSockets specification defines an API that enables web pages to use the WebSockets protocol for two-way communication with a remote host. It introduces the WebSocket interface and defines a full-duplex communication channel that operates through a single socket over the Web. HTML5 WebSockets provide an enormous reduction in unnecessary network traffic and latency compared to the unscalable polling and long-polling solutions that were used to simulate a full-duplex connection by maintaining two connections. The WebSocket protocol was designed to work well with the existing Web infrastructure. As part of this design principle, the protocol specification defines that the WebSocket connection starts its life as an HTTP connection, guaranteeing full backwards compatibility with the pre-WebSocket world. The protocol switch from HTTP to WebSocket is referred to as a the WebSocket handshake.
MQTT - Message Queuing Telemetry Transport
MQTT is a machine-to-machine (M2M)/"Internet of Things" connectivity protocol. It was designed as an extremely lightweight publish/subscribe messaging transport. It is useful for connections with remote locations where a small code footprint is required and/or network bandwidth is at a premium. For example, it has been used in sensors communicating to a broker via satellite link, over occasional dial-up connections with healthcare providers, and in a range of home automation and small device scenarios.
COAP - Constrained Application Protocol
The Constrained Application Protocol (CoAP) is a specialized web transfer protocol for use with constrained nodes and constrained networks in the Internet of Things. The protocol is designed for machine-to-machine (M2M) applications such as smart energy and building automation. Like HTTP, CoAP is based on the wildly successful REST model: Servers make resources available under a URL, and clients access these resources using methods such as GET, PUT, POST, and DELETE. CoAP was developed as an Internet Standards Document, RFC 7252. The protocol has been designed to last for decades. Difficult issues such as congestion control have not been swept under the rug, but have been addressed using the state of the art.
AMQP - Advanced Message Queuing Protocol
The Advanced Message Queuing Protocol (AMQP) is an open standard for passing business messages between applications or organizations. It connects systems, feeds business processes with the information they need and reliably transmits onward the instructions that achieve their goals. The capable, commoditized, multi-vendor communications ecosystem which AMQP enables creates opportunities for commerce and innovation which can transform the way business is done on the Internet, and in the Cloud. AMQP is divided up into separate layers. At the lowest level we define an efficient binary peer-to-peer protocol for transporting messages between two processes over a network. Secondly we define an abstract message format, with concrete standard encoding. Every compliant AMQP process is able to send and receive messages in this standard encoding.
In
REACH we build our system based on above mentioned technologies, so our approach is to use existing and well-designed open standards and protocols so we support all the application level protocols described above on the top of our modern microservice architecture.
Sources:
https://gyires.inf.unideb.hu/GyBITT/08/ch05s02.html
https://www.websocket.org/aboutwebsocket.html
https://coap.technology/
http://www.amqp.org
Advanced document processing by Big Data techniques
To build up a professional document management system is crucial for every organization. It usually provides functions like document storage, document classification, access control, and collaboration. Nice, but is it enough? Can we really use the information stored in these files effectively? In this post, we are going to show how you can gather and use valuable information from unstructured documents by Big Data tools and techniques.
Most of the companies deal with a large amount of unstructured data in various file formats. The most popular types are the different versions of Word, Excel and PDF. In addition, scanned documents and other images are also remarkable. The unified process of these could be a great challenge due to the diverse file types. It is good news that 'processing various data' is one of the main definitions of Big Data (besides 'large volume' or 'fast velocity').
So we have powerful Big Data tools to apply. We can analyze the metadata of documents, get the content in a unified text format even from scanned documents or build up a 'google-like' internal search engine. To develop a custom Big Data application with the previously detailed features, we can use plenty of open source software components. Let’s see a Content Extractor and an OCR solution in details.
Apache Tika
The Apache Tika toolkit detects and extracts metadata and texts from over a thousand different file types (such as PPT, XLS and PDF). All of these documents can be passed through a single interface. This makes Tika useful for search engine indexing, content analysis, translation, and much more. With Apache Tika, we can grab all metadata and text-based content from any popular document type.
Tesseract OCR
Tesseract is an Optical Character Recognition (OCR) engine with support for unicode and the ability to recognize more than 100 languages out of the box. This software released under the Apache License is free, and its development has been sponsored by Google since 2006. We can effectively use it to extract text content from scanned documents or any other images. Nowadays, Tesseract is considered as the best open-source OCR engine, regarding the accuracy of the recognized texts.
Metadata analysis
Besides their effective content, the mentioned documents also contain a lot of metadata. The most common metadata are: author, creation date, last modification date, last modifier, creator tool, language, content type ..... etc. In case of images, we have metadata about the application that optionally modified the original photo and perhaps the exact GPS coordinates of the location, as well. If we can extract these data and store them in a unified way in a database, we will have the capability to run advanced search queries on them. On top of that, we can also create analytics or visualization about our documents (for example, the distribution of 'Creator tools', a number of documents created or modified in a certain period ..... etc).
Build a search engine
It is obvious that the more business documents we store, the harder it is to find the relevant information we are looking for. In this situation, a custom internal search engine could be a very useful tool for the whole organization. To build up a search engine, first we have to process all documents we have, and then grab their content, index and store it in a special database, optimized for quick full-text search queries. In case of scanned documents, it’s needed to apply Optical Character Recognition (OCR) to convert the scanned image in an interpretable text format. After the initial document process, we have to build an automated data pipeline, which will ensure the processing of new or modified documents continuously. During the data process, we can also define keywords, and then tag the given document with the found keywords in their content.
Interactive maintenance guide
In a factory with many production lines, regular maintenance is a general task. However, maintenance manuals are usually not unified. It is sometimes hard to find the relevant documentation for a given machine or part. Furthermore, manuals could be updated regularly so it is important to use the appropriate version.
To support this task, we can build a common interactive maintenance guide for all operating machines in the factory. This guide could provide step-by-step maintenance instructions for every machine and store the previous versions of the documents. In order to implement a system like this, we have to process all available maintenance manuals, find the relevant parts in the document, and load the document into a unified database. The use of this continuously updated database and a well-designed user interface will make the execution of maintenance tasks more effective with fewer faults.
Project methodologies for Industry 4.0
We can see that many factories struggle with implementing successful projects aligned with an Industry 4.0 initiative. Success doesn’t always mean financial return directly. However, it can bring better worker and customer satisfaction, environmental benefits and more: being lucrative in the long term. But anyway, there must be a gain from any project, which in turn will drive your company along the (hopefully long and fruitful) Industry 4.0 journey.
We have seen several good initiatives literally die because of one very important aspect: the lack of a reasonable use case, which would increase the appetite to use big data solutions in the factory. During the last couple of years, we have gained experience at customers and international hackathons. As a result, we have a well-working set of methodologies that helps create value at almost any company.
Not sure if there is value in your use case? Do data pre-evaluation
Many times, companies over-plan the implementation of use cases instead of experimenting with what they have and iterate it until they have the final solution. Are you planning to build an expensive data pipeline for a predictive maintenance system? You had better check the quality of the data and take the first steps with an offline system before building something large.
This is the situation our so-called 'data pre-evaluation' methodology is developed for. In only 10 man-days (optimally lasting about 4 weeks, depending on your availability), you will be able to decide whether to invest in a real-time and precisely built version of the same idea.
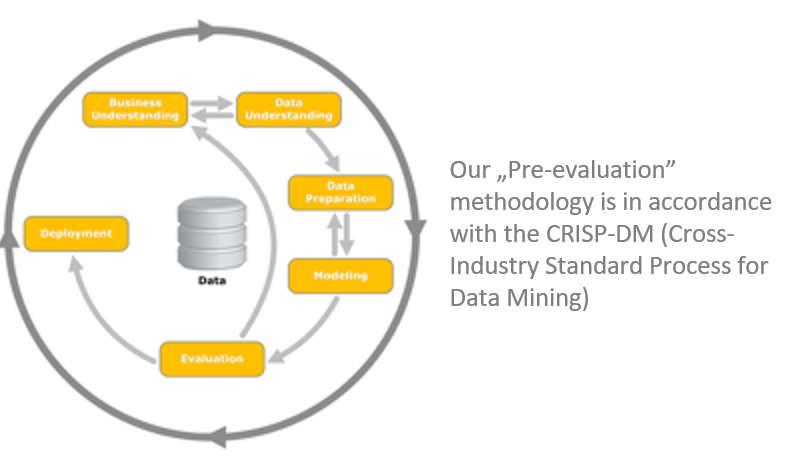
First, prepare and get to know the data by using standard data mining and analysis tools. In one case, our customer had frequent breakdowns on their machining equipment, and process data was present in CSV files. Initially, we cleaned and investigated the data.
Then, we concluded that it would be very likely to build a predictive application by using that data as a training set and calculating the predictions on the live stream data. (Of course, the re-training of the system can happen at any time after the initial training.)
In later phases of the idea implementation (see the proof-of-concept later in this blog post), the predictive application helped reduce downtime significantly.
If engineers and decision-makers understand how powerful a potential application could be (based on its pre-evaluation), they will likely want to build a Proof-of-Concept, which is usually the next step in the iterative process.
Ready to make the next step? Go for the PoC (Proof-of-Concept)
A PoC is meant to be something its name refers to: proving that the initial assumption or concept was right and the solution satisfies the initial claims. That is, the product will meet customer demands; the solution will bring the desired benefits and so on.
We strongly believe that a PoC needs to contain at least 3 use cases (of low-hanging fruit type). This increases the chance that out of these three, at least one will generate a financial return within one year. This is again very important to convince decision-makers inside the factory.
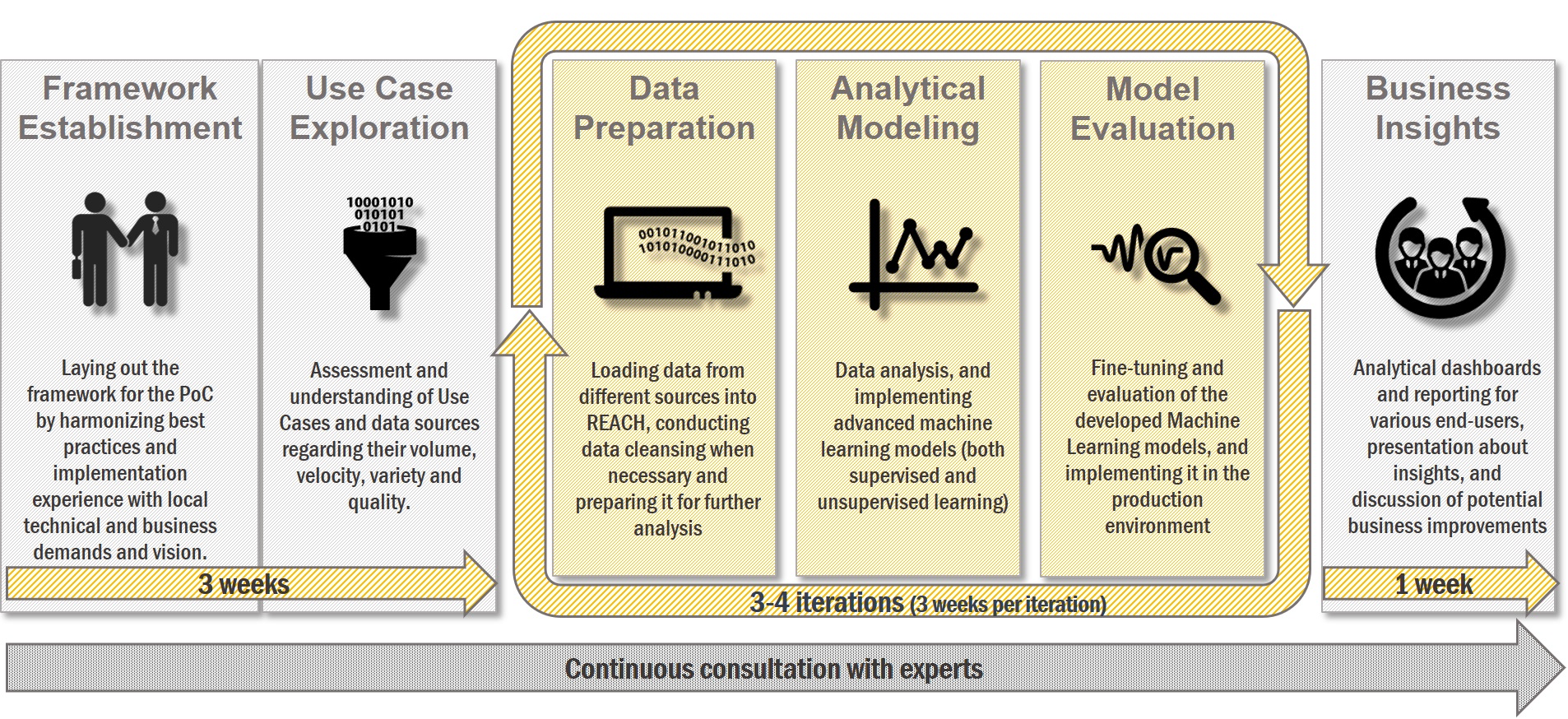
Our agile PoC methodology takes about 3 months, and it has three main phases. In the first iteration phase (3 weeks), we explore the use case more deeply than in the 'data pre-evaluation' methodology as we need to build the foundation for a future production environment. It is followed by 3-4 iterations of modelling and development. This is where data and models are prepared, data pipeline(s) are built, and all of these get evaluated.
At the end, it will take at least 1 week and several discussions with our customers to understand the business insights that we have gained and to evaluate the current and future value of the project. Finally, we report the results to the management and discuss the next steps.
Industry 4.0 roadmap – designing strategies
We have been through numerous project implementations with the players of the manufacturing industry. Sooner or later, every company will realize that either a complete Industry 4.0 or a Smart Factory roadmap needs to be developed. Another solution is when an existing strategy is to be updated or fine-tuned. We can help you identify key areas in the factory where proven Big Data technology can help make production more efficient and profitable and help design the strategy that will result in a Smart Factory one day.
Sometimes you need to spice up the idea
We have learnt a lot during and from
Industry 4.0 hackathons. One of the most important take-aways is that a spectacular demonstration of the use case is necessary to convince decision-makers to start a project, which will be deployed in a production environment later. Using the 'hackathon method' helped us convince key people to continue and profit from the Industry 4.0 roadmap. For example, the spectacular demonstration of a prototype version of the machining use case was enough to prove that it is worth taking the next step towards the system in production mode.
In sum, if you feel that you need to start building an extensive solution or application for a specific problem, you had better run a small evaluation with the data and information you already have (if you are playful, you can even use the hackathon format). And if you need help, we will be glad to help you with our proven methodologies. Get in touch!
Digital Transformation 4.0
Digital Transformation shows how companies can upgrade their operations with technology. People like using this term as something 'revolutionary' a brand-new approach. However, digital transformation is not a new concept. In fact, we are faced with the fourth wave of digital transformation. This applies particularly to the industrial sector, where the digitalization has a long history. Let's look at it briefly.
Digital Transformation 1.0 (1970s): Initial Digitalization.
The whole story began somewhere around the PLCs at the end of the ‘60s. PLC means 'programmable logic controller', which is an industrial digital computer. It has been adapted for the control of manufacturing processes, such as assembly lines or any activity that requires high reliability control, ease of programming and process fault diagnosis. A PLC is an example of a real-time system since output results must be produced in response to input conditions within a limited time, otherwise unintended operation will happen.
Richard Morley is generally known as the 'Father of the PLC', but General Motors delivered the first batch of PLCs in November 1969.
Digital Transformation 2.0 (1980s): Transformation to Paperless Procedures.
In the initial wave, the number of programmed solutions was steadily growing. In the 1960s and 1970s, the development of electronic data interchange systems paved the way for the second wave of digital transformation.
In this phase, computer systems already supported different business activities (e.g. booking, invoicing, ordering and accounting). They enabled different planning and management processes, as well as the coordination of interdependent activities, which are paperless interactions even with external partners. However, to tell you the truth, during the 1980s, paperless office simply meant that all forms of paper (documentation) should be converted to digital format.
Digital Transformation 3.0 (1990s-2000s): Transformation to Automated Procedures.
The trend of using information technology continued during the middle and late 1990s. In particular, automatic identification and positioning technologies were introduced in the mid-1990s to improve the efficiency and safety of operations. The major change was the collection methods, like the adoption of new handling technologies equipped, for example, with sensors.
The automation of certain processes often required the complete redesign of organizational structures, policies and business process activities, as well as efficient information management (at that time, this was called BPR – Business Process Reengineering. Probably, most of you remember it.)
The limitations of static information were still experienced, but higher visibility and different forms of decision support based on accurate data were becoming increasingly important to enhance responsiveness during the operations.
Digital Transformation 4.0 (2010s-): Transformation to Smart Procedures.
The basic idea is to integrate different systems and data silos into one central platform. Based on real-time data, this allows decision-making and ongoing interaction with stakeholders thus being actively involved in the manufacturing activities. Data silos occur for different reasons. The earlier digital transformation waves produced tons of different IT applications. Once a company is large enough, people naturally begin to split into specialized teams in order to streamline work processes and take advantage of particular skill sets.
In the new era, lots of data are still processed in isolated systems. However, in parallel, these are immediately transferred to a central information system in order to explore, analyze and distribute relevant and valuable information over different channels to various targets (humans, systems, machines).
Maybe, the most important part of this phase is the 'rise of artificial intelligence'. Artificial intelligence helps you get the work done faster and with accurate results. A central and intelligent information system will facilitate integration and provide the necessary resources to fulfil the required business agility in a flexible way.
An integrated operation of different, but particularly collaborative systems and devices can be realized in a central solution controlled by AI. This can simultaneously handle past heritage, present urgency and future uncertainty for agile mass customization on the whole value chain. From IT perspective, this needs an IoT platform for integration, as well as for fast and smart processing. That’s what REACH offers. And this is the point where Digital Transformation 4.0 and Industry 4.0 directly meet each other.
Data assets at a factory
Special benefits of IoT and Industry 4.0 for manufacturing companies
Earlier we emphasized that the biggest winners of Industry 4.0 will be companies that rapidly find out how to turn their data into real business benefits. In this article, we will show you why an integrated Internet of Things (IoT) platform is the proper solution.
IoT and 'data revolution' do not disrupt manufacturing businesses, unlike in the case of other industries like telco and retail. Manufacturing companies seek the optimization potential in their data and the intelligence that can be provided to it. The aims are to make production more efficient, reduce scrap and waste, in other words: to support lean manufacturing.
Think about today’s technological advancements in manufacturing compared to the production of the Ford Model T. A lot of machines are automated and most of them collect data. Still, fewer than 5% of the machines in factories are monitored in real time. This is a huge obstacle to full transparency.
Estimating the value of data and the information contained in a project is essential to decide where to implement industry 4.0. Let’s see the major approaches that can be used to determine the value of data within an organization.
Different approaches to measure the value of data:
- Benefit monetization approaches: the value of data is estimated by defining the benefits of particular data products, and then monetizing the benefits.
Let's see an example: a machine starts producing waste from time to time, with no significant change in the operation state. When this happens, the machine is stopped for 20-30 minutes, and the tools get cleaned. If producing waste can be predicted, the operators are able to avoid the problem. This generates savings of 1-hour machine time each day and about 50 waste pieces per day.
In this example, we use data from the machine to predict unwanted events, to avoid them by intervention and to create measurable benefits.
- Impact-based approaches: here, the value is determined by assessing the causal effect of data availability on economic and social outcomes, even within the organization. In addition, (processed) data make daily work more effective and help reduce the frustration of workers fall in this category.
For example, if repetitive, boring work can be automated, it lets workers and analysts do work with more added values and feel happier.
There are further approaches, e.g.: cost-based, market-based, income-based, etc. However, the above two are the most applicable to the manufacturing industry.
Go for the business benefits
The key question is: will the particular data provide measurable and tangible business benefits? The critical first step for manufacturers who want to make use of their data for improving yield is to consider how much data the company has at its disposal.
Most companies collect vast troves of process data, but typically use them only for tracking purposes, not as a basis for improving operations. For these players, the challenge is to invest in such systems and skill sets, which will allow them to optimize their use of existing process information.
The data silo problem
Having data is not enough. Very often, companies' data remain under the control and use of distinct departments, and thus the information flow is blocked. In these situations, we talk about data or even information silos.
A data silo is a repository of fixed data that remains under the control of one department and is isolated from the rest of the organization.
Data silos are huge obstacles if a company wants to make operations more visible and transparent. If you notice that data silos have been developed in your organization, you may want to look for a solution and build bridges between them. In this case, you will probably end up with an IoT platform.
The solution – an IoT platform
An IoT platform like
REACH is basically the nervous system of any factory. It connects different functional units, machines and sensors with humans, it transfers signals in both ways, stores and analyzes data and it must be able to exhibit intelligence to some extent.
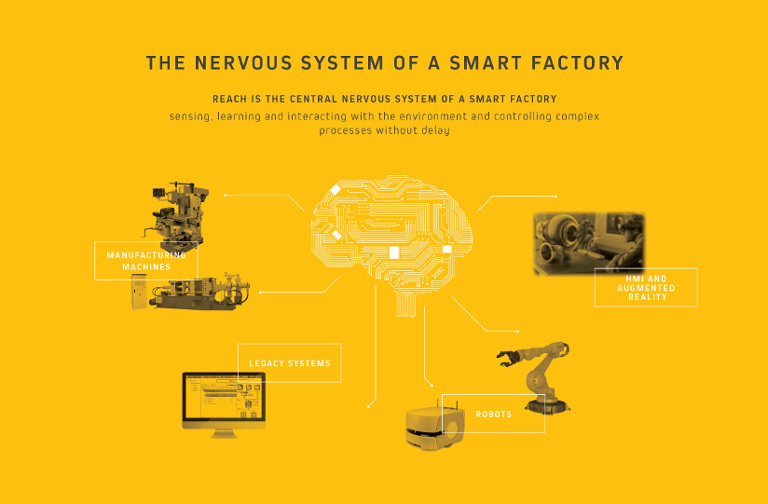
Data must be flowing from different production phases, machines and departments with no friction. Predictive maintenance algorithms need to monitor the whole procedure for the timely alert of the right staff that can prevent or eliminate any failure.
If you want to reach this functionality, a cross-department/ cross-operational IoT platform must be in operation at your company.
This is the preliminary condition of any integrated Machine Learning and Predictive Maintenance solution .
Sometimes, only one missing link between data sources can provide a huge benefit. Imagine that you operate a gluing machine at some point in an assembly process. The adhesion force of the glue varies with time and you don’t know why. There are days when you produce 20% scrap because of insufficient adhesion quality.
Even if you analyze the data collected from a machine, there is no pattern that would imply causality between machine data and the final product quality. At some point, you get the idea of joining the machine data with the factory weather station, and you will find that the gluing quality correlates with air humidity, so you can start solving the problem and reducing scrap significantly.
Implementing a profitable use case
Implementing a use case that provides measurable business benefits is not solely dependent on the data sources and the quality of the data. The ability of creating real value must lie within the organization and require good methodologies.
In our next blog post, we will explain some of these proven techniques that help companies implement successful and profitable use cases and projects.
Data security
A key factor for applications dealing with lots of data – including complex event processing – is security. Nowadays as Internet of Things (IoT) is more popular than ever, one can hear more and more stories about security breaches. Simple internet connected devices are often less secured, and thus more vulnerable to different types of attacks.
Limitations of Fog Computing
REACH uses Fog Computing , which means that none of the data leaves the factory's territory, thus making any external attack impossible. Naturally, this doesn't mean that everything is secured. If hundreds, thousands, or even tens of thousands of employees and vendors can access every data without restrictions, the chance of a potential disaster is excessive. Just think about what could happen if someone deletes all data collected in the past years it doesn't matter if it is intentional or not.
Prevention is better than cure
Most companies only think about security after Armageddon has already happened: a leak or destruction of private data. All such incidents are avoidable with enough care. Fortunately, there are multiple ways to address these problems, and REACH also has these solutions integrated by default.
How can Kerberos help authentication?
Kerberos – developed by MIT – plays a key role in authentication. It only lets people (and services) access data if they can prove their identity. The client authenticates itself at the Kerberos server, and receives an encrypted timestamped ticket-granting ticket (or TGT for short). Whenever it wants to access a new service in the TGT’s lifespan, it asks for a separate ticket for the specific service. Different services are accessible only with these valid tickets, which also have a lifespan, and thus they are unusable after a short period: all tickets are encrypted with AES256, which could take an eternity to brute force with billions of supercomputers.
Improving security by LDAP (Lightweight Directory Access Protocol)
The next level of security is authorization, where rules specify who can do what. Lightweight Directory Access Protocol – shortened as LDAP – is an industry standard for distributed directory access, created by the University of Michigan. Its OpenLDAP implementation is fully open source and integrates well with Kerberos, thus making it a perfect fit for security. It holds all information about users and services, and says which user has permission to access a specific resource.
Preventing data loss by TLS (Transport Layer Security)
However, one piece is still missing: what if fog devices are communicating with each other? They still have to send data across the local network to collaborate, and one could sniff those packets. The solution to this is using Transport Layer Security (TLS), which is a cryptographic protocol. It encrypts the data over the network, and as a result, only the intended recipient can open the messages.
Remember, no matter how tall, spiky or strong the fence is what you have at 95% of your territory’s circumference! Your fence is as strong as its weakest part.
None of the above technologies would be enough alone, but together they form an all-round security layer to protect your valuable data.
The role of Industrial IoT in maintenance and manufacturing optimization
Maintenance is a daily task in factories to keep machines healthy and the whole manufacturing process efficient. The main goal is to do maintenance before a particular machine starts producing waste or even suffers complete failure. It is easy to prove that preventing machines from being stuck means lower operating costs. In addition, it helps keep production smooth and fluent. Still, a lot of factories have a hard time dealing with downtime due to asset failure.
Standard maintenance procedures – Preventive Maintenance (PM)
The purpose of regular care and service done by the maintenance personnel is to make sure that the equipment remains productive, without any major breakdowns. For this purpose, maintenance periods are specified conservatively. These are usually based on data measured by the equipment manufacturer or at the beginning of the operation. However, such procedures do not account for the actual condition of a machine. This can be influenced by different environmental effects – like ambient temperature and air humidity –, raw material quality, load profiles, and so on.
In order to take the ever changing operation conditions into account, condition-relevant data needs to be collected and processed. This is condition based maintenance (CBM). In case of machining it is essential to measure ambient parameters, machine vibration, sound and motor current, which give a picture about the concrete health state of the machine and machining tools. The availability of this data enables making the step towards a more sophisticated maintenance mode: Predictive maintenance.
In order to take the ever-changing operation conditions into account, condition-dependent data need to be collected and processed. This is called Condition-Based Maintenance (CBM). In case of machining, it is essential to measure ambient parameters, machine vibration, sound and motor current, which give a picture about the health state of the specific machine and machining tools. The availability of these data enables you to make a step towards a more sophisticated maintenance mode ̶ Predictive Maintenance.
How to bring maintenance to the next level: Predictive Maintenance (PdM)
Maintenance work that is based on prediction, presumes fulfilling the following requirements. First of all, data collected from the asset should contain the information that shows the signs of an upcoming event. In other words, patterns precisely describing each event should be identifiable in the measurement signals. If this hypothesis holds true, the next step is either to hard-code the conditions indicating any oncoming failure, or to use a machine learning algorithm to identify and literally learn the particular failure mode patterns.
The second requirement comes into picture as soon as the patterns have been identified and the model is capable of predicting an unwanted event soon enough to take action. This requirement addresses the architecture of the system by making the following prediction: “It needs to operate in real time.” The reason is that there are many applications where damage can be predicted only shortly before the event (usually measured in minutes or seconds). Advanced IIoT systems feature real-time operation.
And last but not least, events and data are very complex in manufacturing situations where multiple machines and robots are involved. It means that a group of machines together has an impact on the product quality or operational efficiency of consequential machines. Processing such data requires a system with high computing performance and tools to handle complexity.
The complex data processing and predictive capability of REACH lies in the most advanced Big Data technologies, built-in Machine Learning algorithms and the real-time Fog Computing architecture . The system is capable of learning and distinguishing between failure modes and sending alerts in case of an expected breakdown.
Although Predictive maintenance is a big step for most manufacturers, there is still a next level to go for.
State-of-the-art: Prescriptive maintenance (RxM)
Prescriptive Maintenance requires even more detailed data and a checklist on what actions to take in case of a detected failure mode pattern. Although technology makes implementing prescriptive systems utterly possible, few organizations make it to this point. This step requires very good harmonization between the maintenance and the production departments, a fair understanding of the problem and efficient cross-departmental information sharing. These are the key criteria of a successful Industrial IoT implementation anyway.
Manufacturing companies that make the effort to collect data, analyse problems, understand and prepare their data, as well as to identify the patterns of inadequate operation, can reach the level of a Smart Factory including maintenance operations. In the presented case, not only the checklist is being displayed on REACH UI, but emails and SMS can also be sent to the maintenance personnel and other relevant stakeholders. This minimizes the time required to prepare for taking necessary actions.
Besides using email and SMS alerts, REACH can send status messages to engineers and other personnel even via our chatbot called RITA. Using state-of-the art technology can be fun, too!
IoT Gateways
In our earlier posts, we talked about how to store, process and analyze data. However, we missed a crucial step: How to collect data? For the IoT, an outstanding challenge lies in connecting devices, endpoints and sensors in a cost-effective and secure way to capture, analyze and effectively gain insights from massive amounts of data. An IoT gateway is the key element in this process. Below, we are going to describe why.
The definition of an IoT gateway has changed over time with the developing of the market. IoT gateways function like bridges – just like traditional gateways in networks. Indeed, they bridge a lot by being positioned between edge systems and our REACH solution.
IoT Gateway market
IoT gateways fulfil several roles in IoT projects. IoT gateways are built on chipsets that feature low-power connectivity and may be rugged for critical conditions. Some gateways also focus on fog computing applications, in which customers need critical data so that machines can make split-second decisions. Based on this, IoT vendors can be divided into three groups. Suppliers who only provide hardware (Dell) belong to the first group, companies who focus on software & analytics (Kura, Kepware) are in the second one, while the third group contains end-to-end providers (Eurotech).
Our IoT Gateway solution belongs to the software & analytics group, which is an OPC client (communicating with an OPC server). OPC is a software interface standard that allows the secure and reliable exchange of data with industrial hardware devices.
What are IoT Gateways?
Gateways are emerging as a key element of bringing legacy and next-gen devices to the Internet of Things (IoT). Modern IoT gateways also play an increasingly important role in helping to provide analytics. Thus, only the most important information and alerts are sent up to the REACH to be acted upon. They integrate protocols for networking, help with the management of storage and analytics on data, and facilitate secure data flow between edge devices and REACH.
Mainly in Industrial IoT, there is an increasing movement towards the fog as is the case with many technologies
Intelligent IoT gateways
With fog computing (and the movement to the edge overall), we really enter the space of what is now known as an intelligent IoT gateway. In the initial and more simple picture, an IoT gateway sat between the sensors, devices and so forth on the one hand and the cloud on the other. Now, a lot of analytics and filtering of information is increasingly done closer to the sensors through fog nodes for a myriad of possible reasons as explained in our article on fog computing. The illustration below shows where the intelligent IoT gateway (and soon all of these will be intelligent) sits in an IoT architecture.
(img source: https://www.postscapes.com/iot-gateways/ )
Machine Learning
Machine Learning is a process of making software algorithms learn from huge amounts of data . This term was originally used by Arthur L. Samuel, who described it as “… programming of a digital computer to behave in a way, which is done by human beings …”.
ML is an alternative way to build AI with the help of statistics. The aim is to find patterns in data instead of using explicitly hard-coded routines with millions of lines of code. There is a group of algorithms that allows you to build such applications, which can receive input data. In addition, these can predict an output depending on the inpu t.
ML also can be understood as a process, when you 'show' tons of data – texts, pictures, sensor data – to the machine with the required output. This is the training part. Then you 'show' a new picture without the required output, and ask the machine to guess the result.
Use cases
Machine learning has grown to be a very powerful tool for solving various problems from different areas, including:
- text processing – for categorizing documents or speech recognizers (chatbots);
- image processing – for training an algorithm with hundreds of thousands of tagged pictures to be able to recognize persons, objects, etc.
However, from our point of view, the more important use-cases are those where factory machines and processes are involved. In this case, we have to collect, assort and store many different sensor readings from various manufacturing robots. We intend to train our machine learning solutions for different purposes like the prediction of machine failures.
Before the training, different tasks have to be done:
- data preparation, for example, filling or throwing out empty cells, data standardization, sorting important features etc;
- training, which involves feeding the cleaned data to the algorithm in order to be able to adjust itself;
- measuring our solution and improving it with new data and parameters.
For these purposes, a data pipeline should be built using the same processes for the newly arrived data as in the training state. With REACH, we are able to do each of these tasks data preparation, training and building the data pipeline easily and in a user-friendly way through the UI. Thus, we will have useful solutions, which will result in downtime and cost reduction, as well.
Machine Learning within REACH
We offer solutions for many different problems occurring during the lifetime of a machine learning project. We have different tools for different roles. We provide an easy and simple graphical UI with pre-configured models, which helps you focus only on the data and the pattern behind it.
Of course, with this approach, you will also be able to tune the model parameters and compare them to find out which parameters are more suitable for your application.
REACH is also perfectly suitable for developers who want to build their own solutions.
With an embedded Jupyter Notebook, users are able to build any model with different technologies including scikit-learn, Spark ML lib, TensorFlow, etc. These models could be deployed to a single machine or distributed to the cluster to reach the best performance and scalability.
Towards the future
Machine learning as a term is really pervasive today. However, it is often used in the wrong way, or it is mixed up with AI. or with deep learning – our following blog post topics.
With this introduction, you could get a little insight into this technology. Our aim was to provide you with a general picture on how to build applications that are able to improve their performance - without any human interaction - using data analysis and the feedback of performance.
Hybrid Architecture
Modern data lakes or classic storage layers?
As we described in our previous blog post, Data Lake for Big Data is a required technology to cover all needs of Industry 4.0. But what about your classic data? Should you transfer them to a data lake? Do you have to redesign all your applications and processes to use a new storage layer?
The answer is definitely not. You do not need to discard your classic databases, systems and processes that use them. According to our terminology and best practices, a classic database engine can operate in smooth symbiosis with the modern Big Data Lake System approach. This only requires a well-designed architecture. However, this is not easy to create.
How can you combine different datasets?
Our experts designed REACH to be ready to handle such situations. We call it a hybrid architecture, where each data goes to its proper place. Some data should be stored in the data lake system, but some of them should go to the classic storage layer.
REACH is designed to be ready to combine different data sources both on process and analytical levels. Thus, at the end of an analysis, you will not be able to distinguish whether a particular data came from the classic layer or from the Big Data Lake.
We firmly believe in using the proper storage technique. According to our approach, each data should go to its proper storage layer. Thus, we cannot advise you to use only one type of storage for all your data.
Further advantages of REACH
Going further into the implementation of this technology, our experts designed REACH with the aim of handling multiple storage layers. However, this implies not only choosing between the Big Data layer and the classic layer.
We suggest that the storage techniques used inside the data lake should be different from the ones in the classic storage layer. A good example for this is when Kudu, HBase and HDFS operate next to each other. This results in extending the storage techniques from the classic layer. Here, the techniques of relational database storage and standard file storage are mixed. This is why we cannot say that one database engine is sufficient.
REACH was designed to support the above multi-storage approach with the aim of getting the maximum out of your data.
Data Lake
In the world of Big Data, traditional data warehouses are not sufficient anymore. These are not enough to support the requirements of Industry 4.0 technology level or to become the foundation of true real-time solutions.
While traditional data warehouses can only offer structured data storage, Data Lake can provide a solution for maintaining the original format and state of the data. Moreover, it can also provide real-time access to them.
The greatest advantage of Data Lake is that it is capable of storing a tremendous amount of data while preserving the raw format in a distributed, scalable storage system. Therefore, Data Lake can store data coming from various data sources, and thus it is adaptable for future requirements. The result is such flexibility, which cannot be provided by the current data warehouses.
What can a Data Lake offer?
The concept of Data Lake enables factories to fulfil the requirements of Industry 4.0. It also makes data generated during production accessible for other participants in the production line. In addition, this can be achieved in the swiftest, smoothest way by the Complex Event Processing method, which was introduced in our previous blog post. Data is stored in its original raw format, and thus there is no data transformation that would slow down this process.
For this reason, REACH has put Data Lake in the heart of its architecture. In this way, we contribute to the competitiveness of our partners. Without the modernization of the storage process, neither real-time analysis nor automations would be possible.
Cut your costs by Data Lake!
Companies that seek to utilize machine learning methods need to possess a wide range of data sources to provide sufficient amounts of data for the algorithms. Cost is also an important element. In case of a Hadoop-based Data Lake which utilizes well-known big data techniques storage costs are minimal compared to a standard data warehouse solution.
This is because Hadoop consists of open source technologies. Furthermore, due to the distributed setup, its hardware requirement is also lower, so it can be built even on commodity hardware.
Should data warehouses be replaced?
During the design of REACH architecture, integrability was one of the main aspects. Therefore, REACH offers interfaces for connecting to various data sources and applications. See our upcoming blog post of hybrid architectures!